Table of Contents
Introduction
Radio-Frequency Spectrum - AM/FM, TV, and Cellular
RF Propagation Measurements
The Longley-Rice RF Propagation Model
Comparisons Between Model and Measurement
RF Propagation Model Limitations
Climate Measurements and Modeling
Climate Change Model Limitations
Conclusion
References
Introduction
I was a research scientist for 31 years at both the National Institute of Standards and Technology (NIST) and the Institute for Telecommunication Sciences (ITS) both located at the Department of Commerce Boulder Laboratories in Boulder, CO. I worked in many different research areas during my career, but my main focus was on measurements and measurement uncertainties.
I want to help people understand how models are developed from scientific observation and the limitations of those models in explaining natural phenomena. I will start with the measurements and modeling undertaken for radio-frequency (RF) propagation of radio signals. Remember the ads, “Can you hear me now?”
I will then move onto the variables that would need to go into modeling the climate and how we can understand just how difficult a task this is to undertake.
Radio-Frequency Spectrum - AM/FM, TV, and Cellular
The radio signals we use to communicate occupy various segments of the electromagnetic spectrum from 9 kHz up to 300 GHz. They are managed by the Federal Communications Commission (FCC) for frequencies used by commercial entities, and the National Telecommunication and Information Administration (NTIA) for frequencies used by Federal Government entities. Frequencies are allocated depending on what they are used for and how that frequency will be used for communication.
Figure 1 shows all of the frequencies allocated for commercial services and federal services and is known as the United States Frequency Allocation Chart.
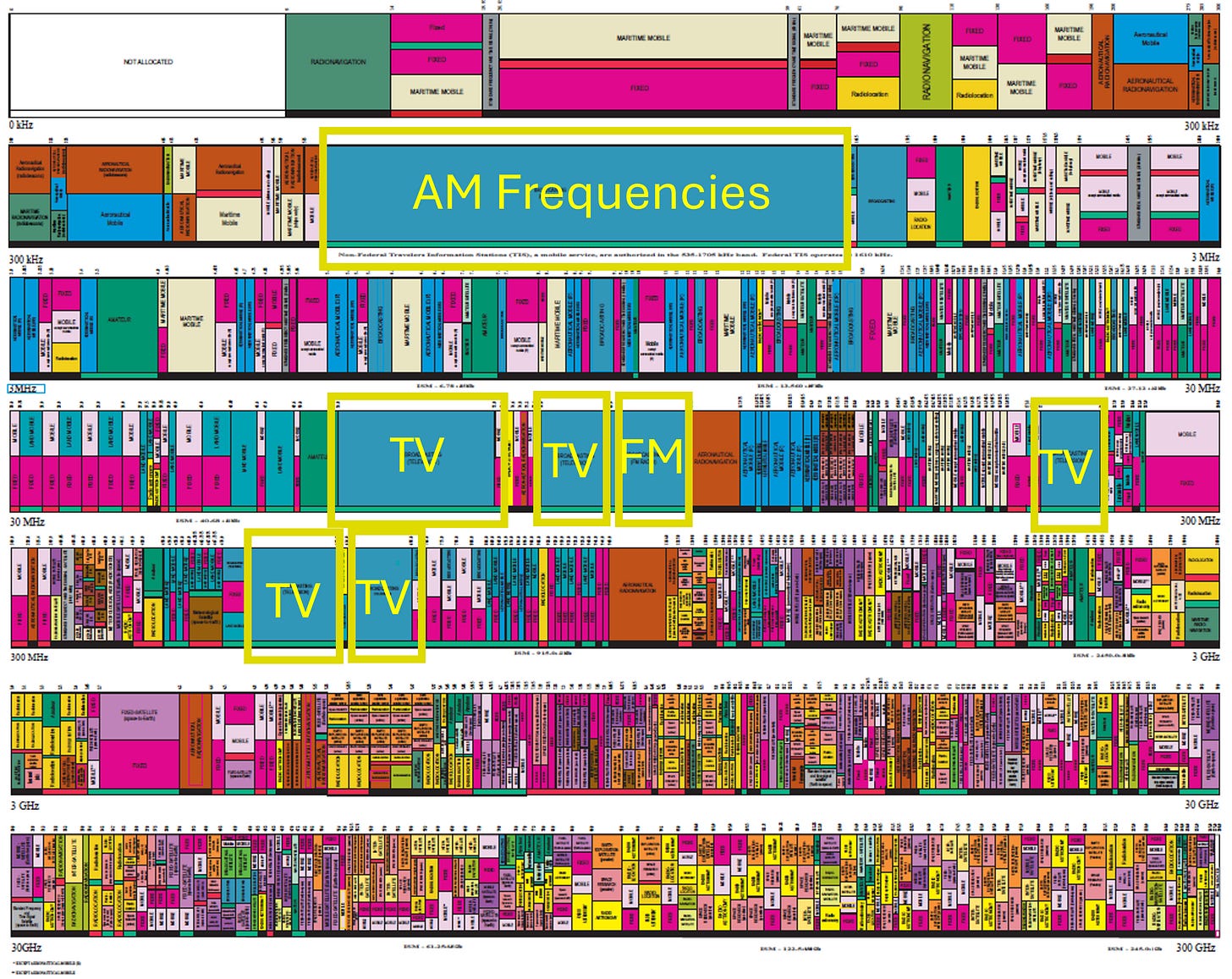
Amplitude Modulation (AM) radio stations operate from 540 kHz to 1700 kHz and the Frequency Modulation (FM) radio stations operate from 87.8 MHz to 108.0 MHz. The AM radio band, the television (TV) bands, and the FM radio band are shown by the yellow squares.
When I worked at NTIA/ITS, we worked with a few of the cellular/wireless carriers to expand their frequency band from 1710-1755 MHz into the Federal Government frequency band from 1755-1780 MHz so that the cellular/wireless carriers could provide more capacity to users. These frequency band allocations are shown in Figure 2, however they do use other parts of the frequency spectrum.
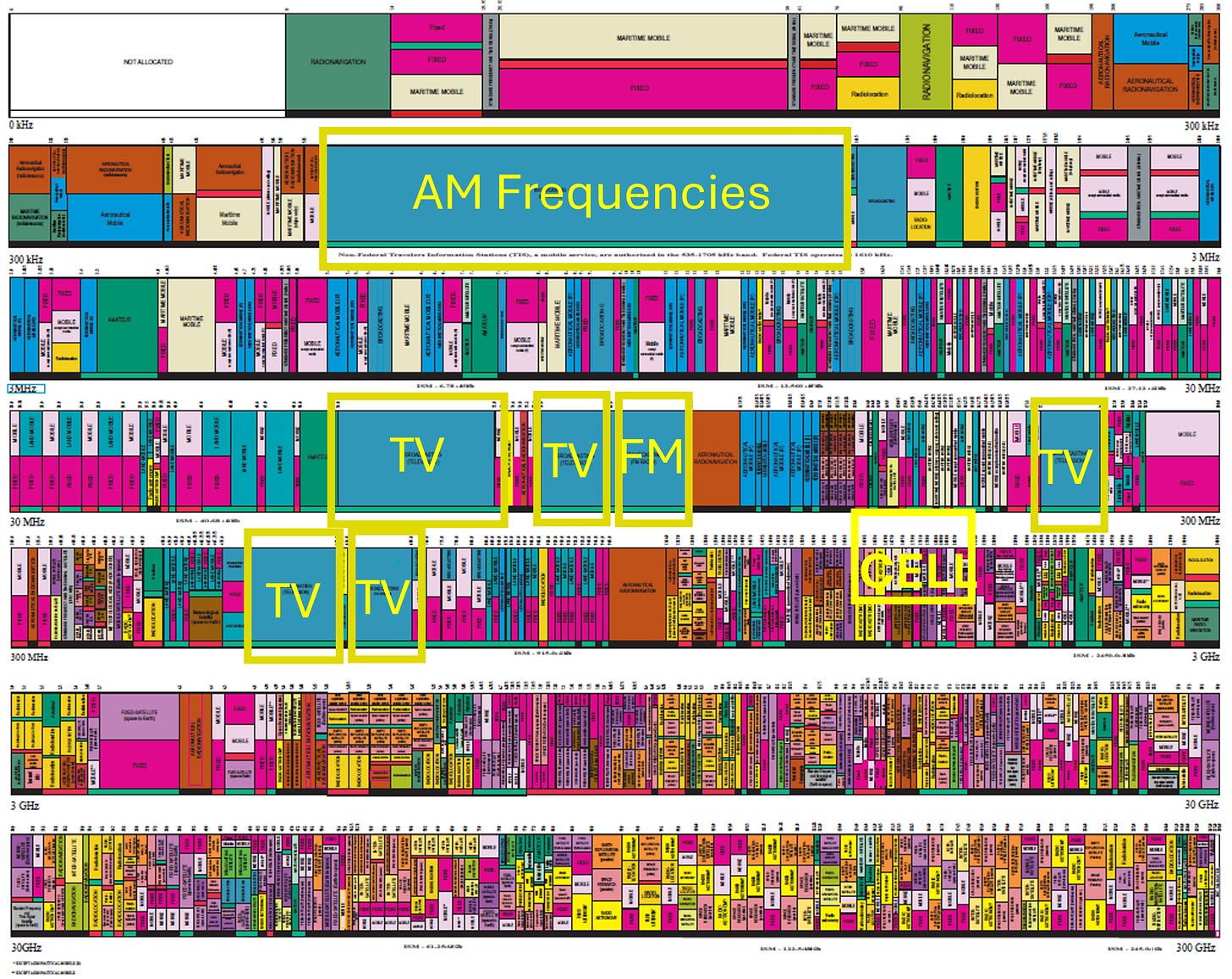
The federal government asked ITS to get involved in a series of RF propagation measurements in the cellular RF bands. These measurements were to be used to develop newer RF propagation models to determine if commercial and federal systems could coexist in a given frequency band. Our measurements were similar to those used by the cellular/wireless carriers, but using different equipment. We worked with the cellular carriers to develop measurement plans and ITS worked to develop and verify a measurement system that was both accurate and repeatable.
Any good scientist will take their measurement instrumentation and test it using reliable standards and procedures. We completed these verification measurements before using the system for the cellular/wireless carriers. References applicable to these measurements are given in the Reference Section at the end of this article.
RF Propagation Measurements
I am going to discuss, at a very basic level, how RF propagation measurements are made. This is the same method used by the cellular/wireless carriers although the instrumentation is different. If you want a technical explanation of the equipment and how the systems were tested, I provide references in the Reference Section at the end of this article.
I will discuss only one location where measurements were completed [1]. This particular set of data was used to understand a very popular RF propagation model which is used to understand how signals lose energy as they travel from the transmitting antenna to the receiving antenna. With that brief introduction, let’s look at how RF propagation measurements are made.
We made measurements in downtown Los Angeles, CA because there is actually a lot of terrain variation between the transmitting antenna and the receiving antenna. We began by placing the transmitting antenna, which is similar to a cell-phone tower, up on an overlook in Griffith Park. The transmitting antenna is shown in Figure 3.
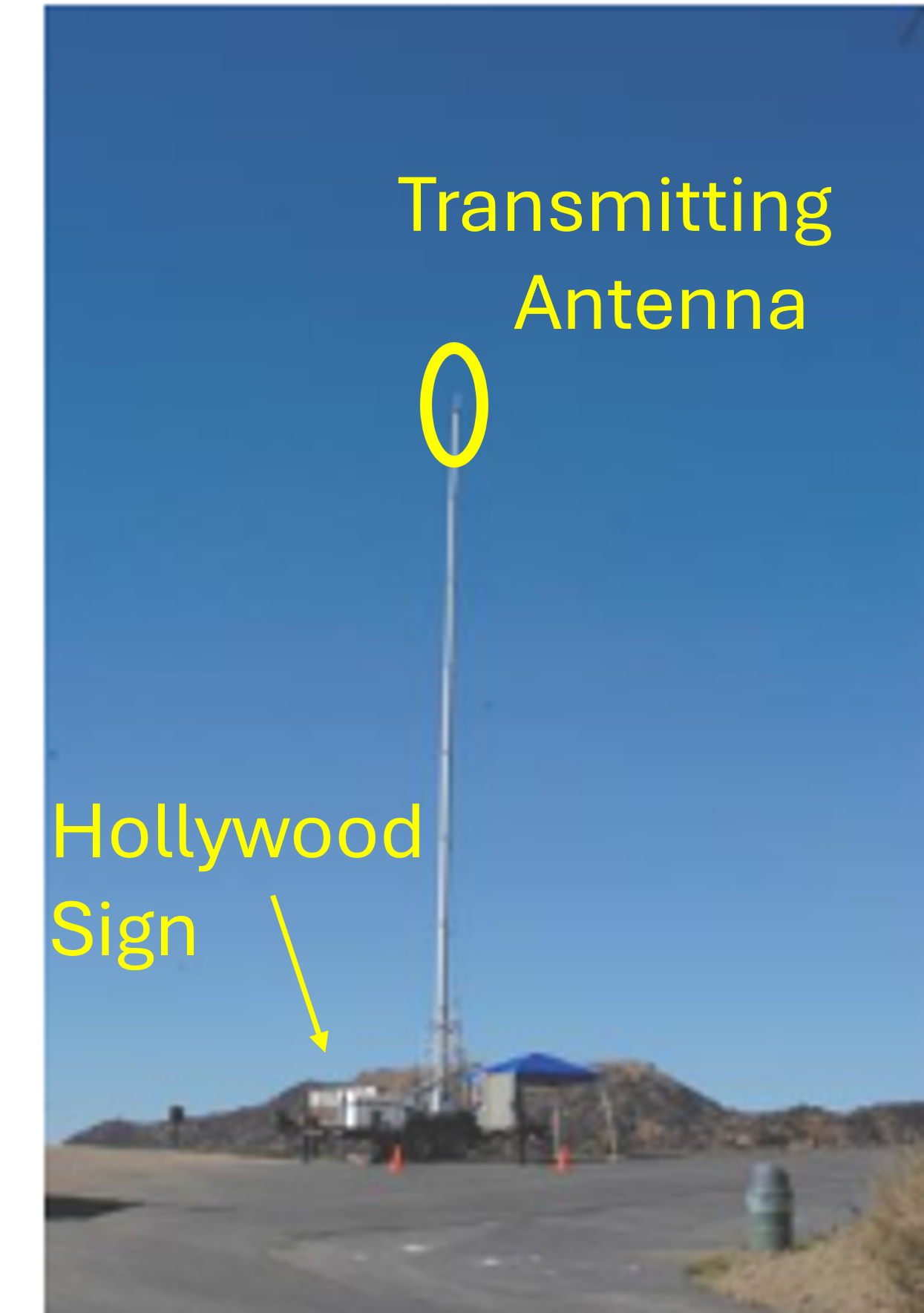
Details including the global positioning system (GPS) location and the elevation of the transmitting antenna are included in the research paper. The receiving antenna was mounted on a van with the equipment used to measure the RF signals on the inside of the van. A picture of the receiving van is shown in Figure 4.
The receiving van was driven along certain roads in Los Angeles to collect data and compare it with the propagation model discussed in the following section. The measurement drive route started near the transmitting location, traveled down the hill, through residential and commercial areas of Los Angeles and into the downtown area and then drove back to the transmitting antenna. We mapped out this route to look at the effects of how signals propagate along roads parallel to the transmitting antenna, how residential and commercial areas affect how the signal travels, and what happens to the signal in areas of tall buildings such as those located in the downtown area. Once the measurement was completed we plotted the RF signal strength along the drive route as shown in Figure 5.
Let’s identify a few points in this image. The first is the transmitting antenna location shown by the red star in the upper left of this image. Downtown Los Angeles is located in the bottom right of this image. The legend shows the Basic Transmission Gain (BTG), which is a related to the signal strength arriving at the receiving antenna and is plotted along the drive route in Figure 5. Pink dots show the highest received signal levels and as we can see from this image, these occur along roads that are inline with the transmitting location. The areas of lowest signal strength are shown by the blue dots and occur in the Downtown Los Angeles area. In this area, there are many tall buildings that block and interfere with the RF signal. The black and white XX’s denote locations where we will compare the RF propagation model with the measurements and try to determine where the model works well and why and where it doesn’t work well.
The Longley-Rice RF Propagation Model
The Longley-Rice RF propagation model, also known as the Irregular Terrain Model (ITM), was developed in the 1960s. This propagation model uses physics to study how terrain (hills and mountains) interfere and reduce an RF signal as it propagates between a transmitting antenna and a receiving antenna. This model does not take into account any RF signal losses due to vegetation, such as trees, or man-made structures like buildings. The model uses measurements of the terrain elevation taken using various scientific methods. There is a lot of detailed information regarding data collection for each of these terrain databases and I will try to provide a simple explanation of how the data was acquired and what is included in the data.
Shuttle Radar Topography Mission (SRTM) - mapped using radar technology in which radar signals were sent to the earth and signals bounced off the top of trees buildings and land. In some of these datasets the buildings and trees were processed out of the dataset, but may retain residual errors. The elevation data is plotted on a 90-meter grid (about 300 feet) which means there is one data point every 300 feet with no information on terrain between these points. Will be denoted as SRTM-90m.
United States Geological Survey (USGS) National Elevation Data (NED) - this dataset used the terrain contours developed by the USGS which was a meticulous mapping of the terrain. These contours are similar to those used in topographic maps. Additional information and artifact removal makes this one of the most accurate terrain databases available. We used a 30-meter grid size (about 100 feet). Will be denoted as USGS-NED-30m.
Light Detection and Ranging (LIDAR) Digital Elevation Model (DEM) - The Army Geospatial Center made these measurements in a similar fashion as the SRTM measurement campaign, except they used light to map the reflections from the trees, buildings, and land. Because the wavelength of light is smaller than the wavelength used for radar mapping, there is more detail in the measurement. We used both a 30-meter and a 10-m (30 feet) grid size for our analysis. will be denoted as LIDAR-30m and LIDAR-10m.
Figure 6 shows the measured basic transmission gain (or signal strength) versus elapsed time in seconds along the drive route.
In Figure 6, the black x’s are the RF signal measurements collected by our system in the green van, the red circles show the data generated by the Longley-Rice model using the USGS-NED-30m terrain database, and the blue line is a physical model based on the expected RF signal that would arrive between the transmitting antenna and the receiving antenna with nothing to interfere with the RF signal between the two antennas.
Quick Note: the unit for Basic Transmission Gain is noted as a dB. For a good explanation of what this means, check this website out. But quickly, let’s say I transmitted energy at 1 Watt and my signal at the receiving antenna was -120 dB, that means the 1 Watt RF signal strength was received with a value of 0.000000000001 Watts. That is a very small signal to receive and requires a very sensitive instrument.
Notice that sometimes the red circles (the model) lie on top of the blue line, these are locations where the model thinks there were no physical obstructions between the transmitting and receiving antenna and if the measured data (the black x’s) also touch that blue line, then the measurements also confirm there were no obstructions between the two antennas. In locations where the model and the measurement do not agree, that means the model is either missing the terrain obstruction (the red circles are above the black x’s) that resulted in the RF signal loss or the model predicted too much loss (the red circles are below the black x’s).
To understand the areas where the measurements and the model do not agree, we ran the model and looked at the terrain profile along the path between the transmitting and receiving antenna. We began our investigation using the USGS-NED-30m terrain database. One of these terrain profiles is shown in Figure 7 which corresponds to the right-most, black X in the upper left of Figure 5.
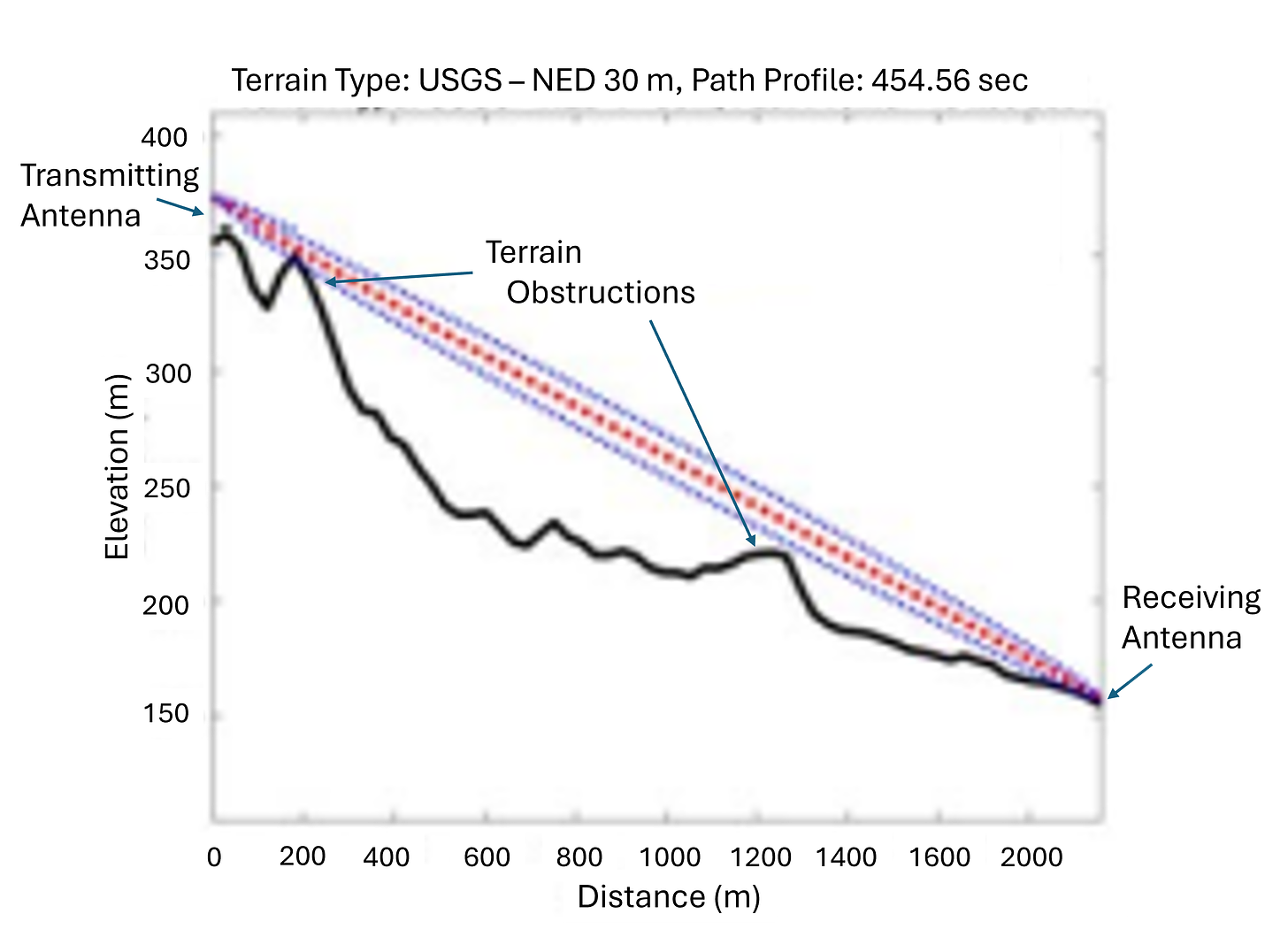
In this figure, the thick black line shows a 2-D profile of the terrain from the transmitting antenna to the receiving antenna, which are denoted respectively. The terrain obstructions are identified in the figure. The red, dashed line shows where the line-of-sight (LOS) between the two antennas is located. This path would be the predicted value shown in Figure 6, where nothing contributes to signal loss except for the physical loss of signal. In other words, if your cellular/wireless phone was in an area with no trees, no hills, and no buildings, you would be able to clearly hear the person on the other end of your phone call.
If any of the terrain profile crosses the red line, then the model calculates the RF signal loss based on a physics-based diffraction analysis and calculation from the terrain details. These terrain obstructions may be a possible cause of dropped calls when you are on a call from your cellular/wireless phone. The more the obstruction crosses the red line the more RF signal loss there will be. The blue, dotted lines show where the major concentration of RF signal or energy is located as the RF signal propagates from one antenna to another.
Comparisons Between Model and Measurement
In this section we are going to look at the Longley-Rice RF propagation model and how the predicted RF signal loss changes as the terrain database that generates the terrain profile shown in Figure 7 changes. We will also compare predictions for the Longley-Rice RF propagation model and the measurements for the black X point in Figure 5. The output from the model calculates the terrain losses for the four different terrain databases discussed above.
A terrain profile is just a cut through the terrain from the transmitting antenna to the receiving antenna. If we zoom into this location in Figure 5, we can show the cut, for the four terrain profiles, using a white, dashed line as shown in Figure 8.
The terrain profiles generated along this path for each database are shown in Figure 9.
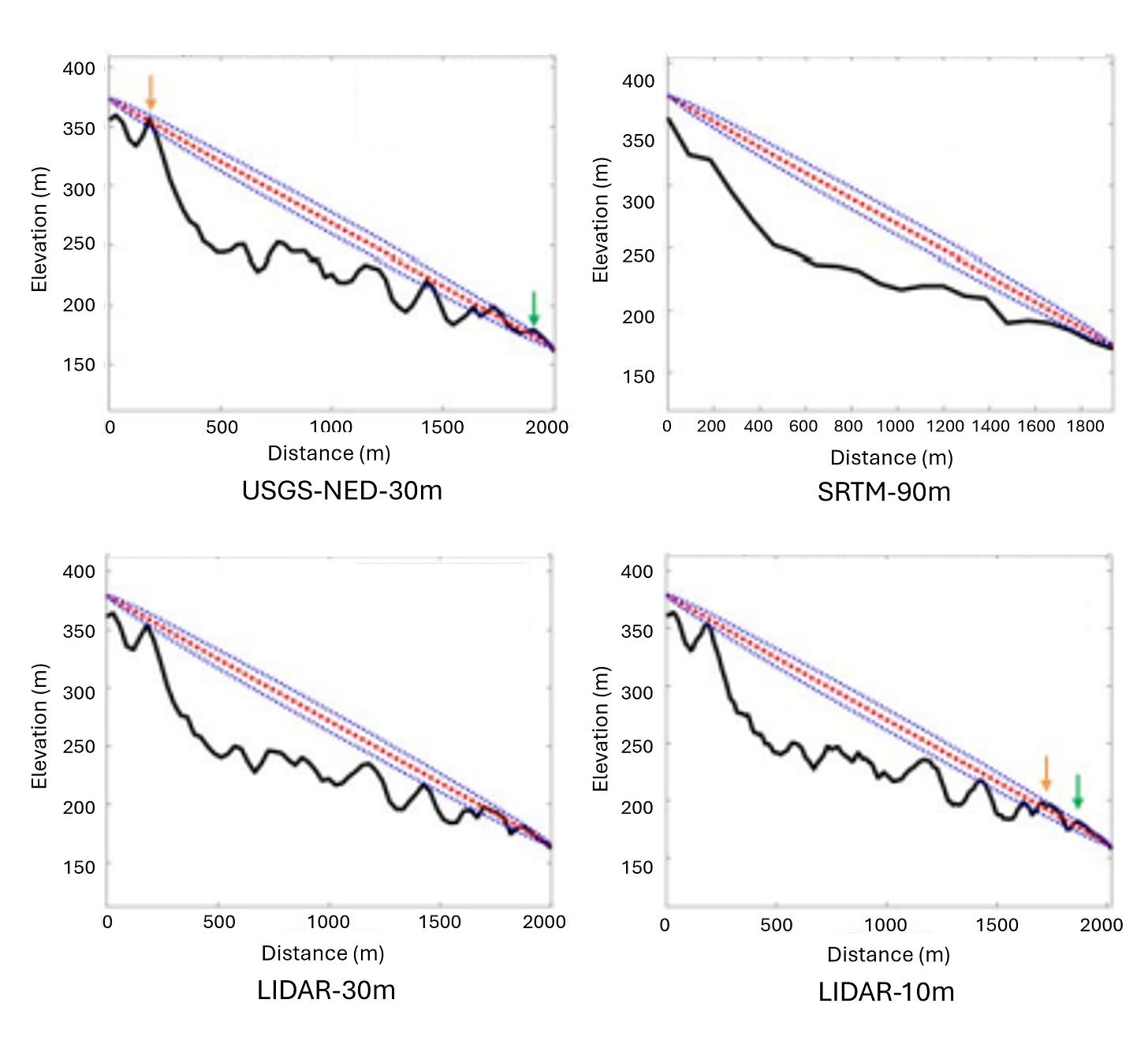
Although the path extracted from each database uses the same GPS coordinates, each terrain profile is distinctly different. Let’s begin by taking a look at the SRTM terrain profile in the upper right of Figure 9. Remember that the SRTM terrain only shows terrain every 90 m or approximately 300 feet which will miss a lot of terrain variation in between those 90 m points. The 30 m LIDAR and USGS-NED datasets look at 30 m intervals, so more terrain variation is included along these paths. Finally the 10 m data includes even more points in between the antennas. If we look at the terrain difference between the SRTM-90m data and the USGS-NED-30m data, we come up with the image shown in Figure 10. The black trace shows the USGS-NED-30m terrain profile and the orange, dashed trace shows the SRTM-90m terrain profile.
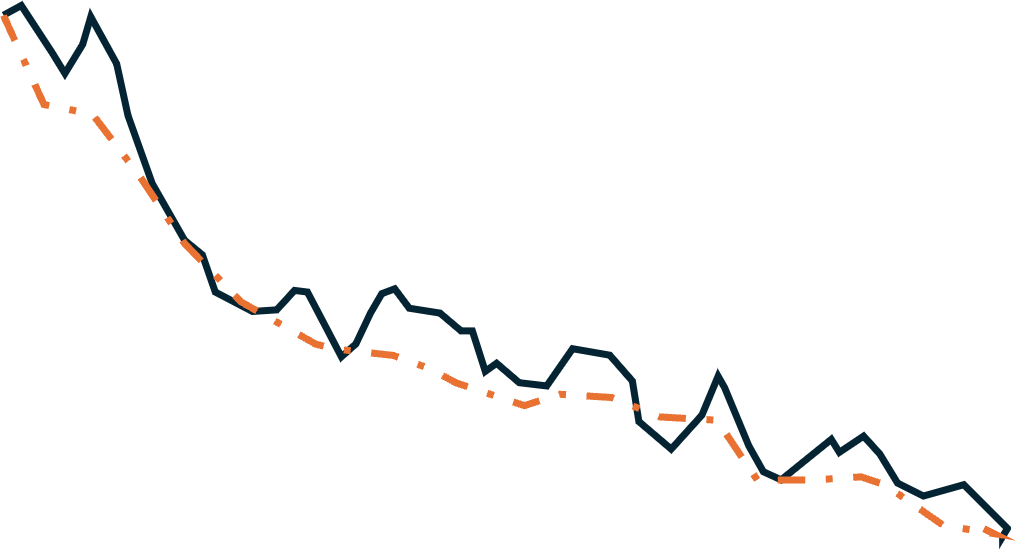
When we only look at terrain every 90 m (orange - SRTM-90m), we lose resolution and miss the important features in the terrain profile which will affect the model. Let’s add the LIDAR-30m data (purple) which captures terrain detail every 30 m or approximately 100 feet. This is shown in Figure 11.
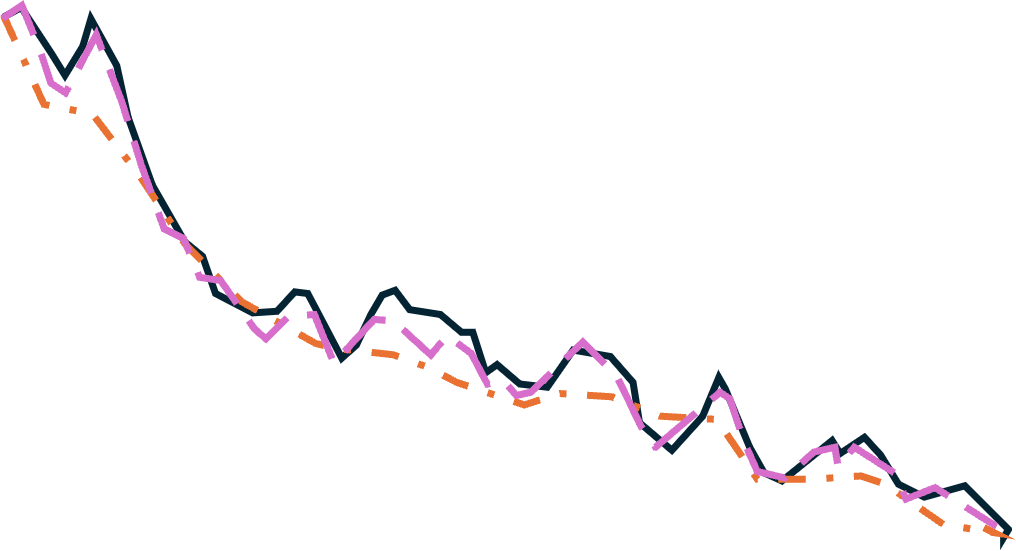
The LIDAR-30m terrain data (purple) is pretty good at locating all of the prominent terrain features, but the elevations do not match precisely the height of the terrain features in the USGS-NED-30m terrain data (black). Let’s add another profile from the LIDAR terrain database, but we’ll pull the data every 10 m. This is shown in Figure 12.
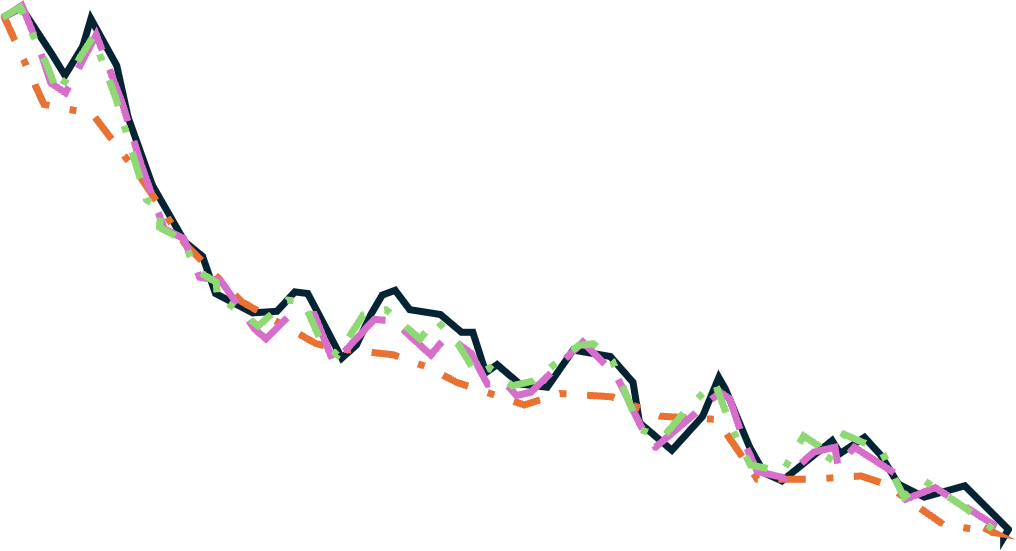
The LIDAR-10m terrain profile (green) adds a few more details to the LIDAR-30m terrain profile (purple), but still shows differences to the USGS-NED-30m terrain profile (black). Now consider the differences in elevations and if the model depends on any part of the terrain profile crossing over the red, LOS line. That would definitely change whether the model predicted RF signal loss which could explain why the measured data showed either no or lower RF signal levels.
Looking back at Figure 9, in two of the graphs there are orange and green arrows. These are locations where the model determines that the terrain profile crosses the red, LOS, dashed line at that location. The model will then compute the RF signal losses based on the amount of terrain that is above the LOS line. From Figure 9, we see that the amount of terrain crossing over the LOS line, is not the same for each terrain profile, this will result in inaccuracies in the prediction for terrain losses.
How inaccurate are these predictions? We will explore that question in the following graph and table.
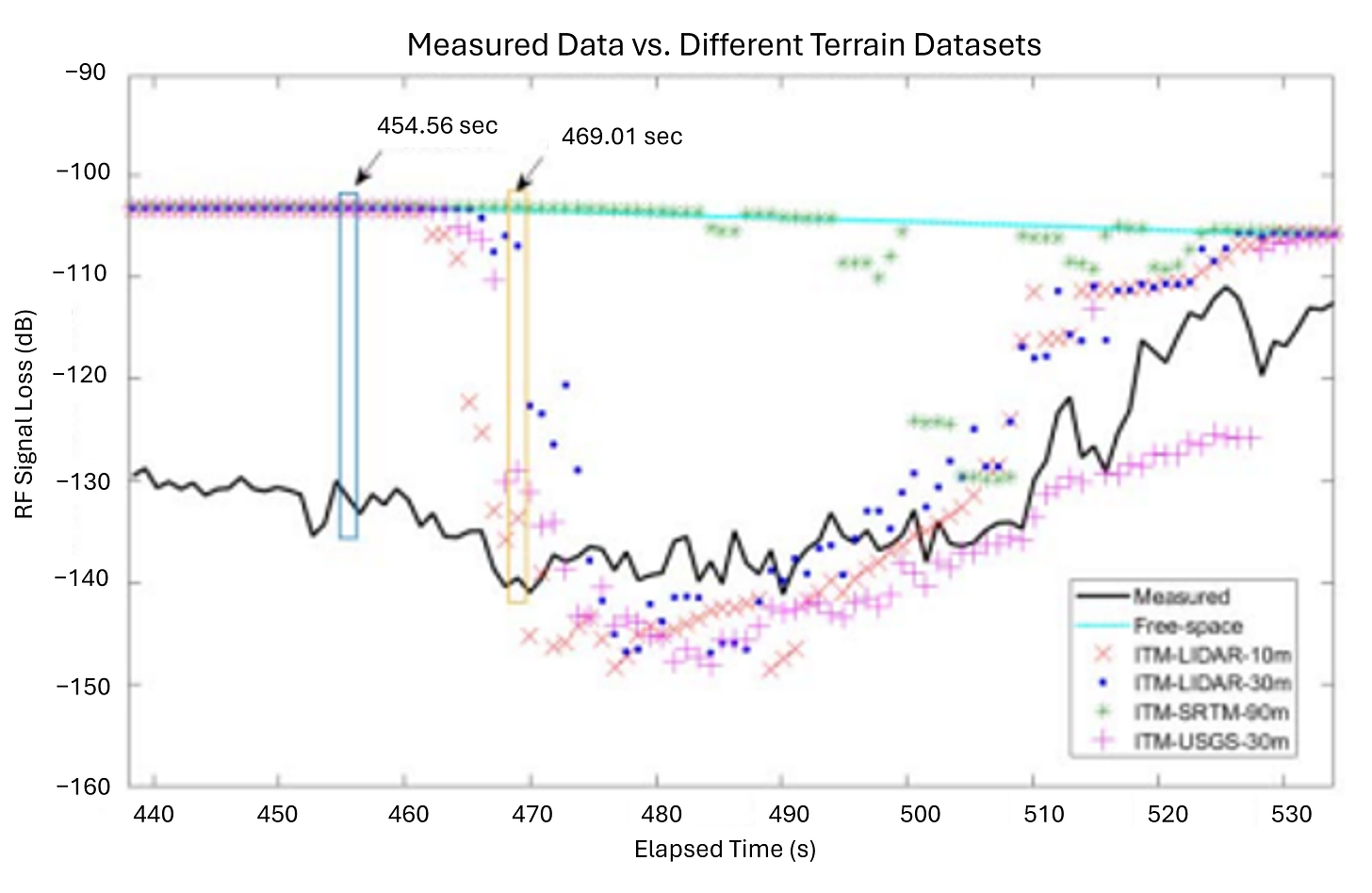
Figure 13 shows the predictions made by the Longley-Rice RF Propagation Model (ITM) for each terrain database. The orange box encompasses the predictions and measurements for the terrain profile considered in Figures 9 - 12. The measured data is the black line, the USGS-NED-30m prediction is shown by the pink plus signs, the SRTM-90m data is shown by the small green plus signs, the LIDAR-30m prediction is shown by the blue dots, and the LIDAR-10m prediction is shown by the red X’s. None of these predictions match the amount of RF signal loss that the measured data shows. The SRTM-90m data thinks there is no terrain as discussed above and shown in Figures 9 and 10. The LIDAR-30m show only a little loss and the LIDAR 10m data shows a large amount of loss. The USGS-NED-30m data shows a value of loss somewhere between the LIDAR-30m and LIDAR-10m data. For those of you who prefer numbers in tables, here are the predicted values for each of the terrain databases, again we are looking at the orange box.
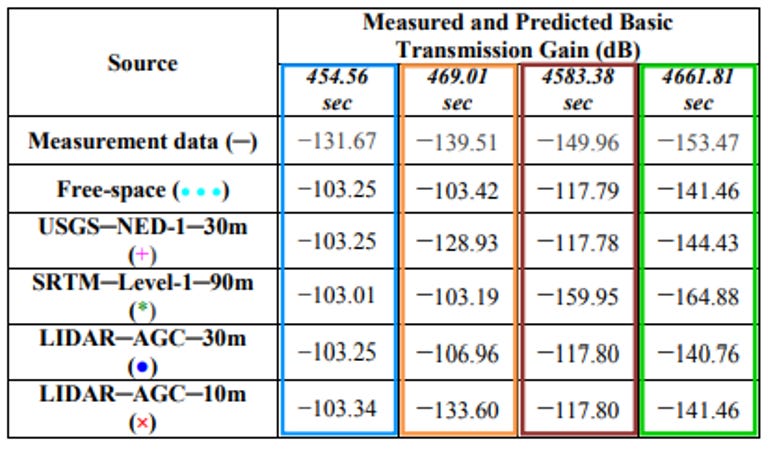
Table 1 shows the measured and predicted RF signal losses for the four terrain databases. The values shown in the orange box correlate to the orange box located in Figure 13 and the terrain profiles shown in Figures 9-12. Free-space loss is just the RF signal loss predicted which would result if there were no physical obstructions in the path between the transmitting and receiving antennas. The measured data shows the highest RF signal loss, The prediction for the SRTM-90m data shows a little more RF signal loss than free-space RF signal loss. The other predictions are in between these two values as discussed in the paragraph above.
RF Propagation Model Limitations
So why are the modelled predictions so different than the measurements, and which one is correct? The answer is that we accept the measurement as ground truth and realize that the terrain-only model does not yet take into account all of the variables needed to be considered in a model.
Let’s talk about the measurements first. We did everything we could to test the system and verify it’s accuracy and repeatability. We also made sure the data processing was accurate and gave us a valid, measured result. The raw data, taken by the instrument, is a series of 0’s and 1’s or digital data and needs to be converted so that it can be compared to the values predicted by the model. As good as the instrument is, it still has limitations. The measurement industry continues to refine and make instruments more accurate. The effort to improve instrumentation is an ongoing process and every generation of instruments are better than those used in the past.
A model is a simplified representation of the real world. For example, an RF signal propagates in a three-dimensional (3-D) environment, but our model only takes into account a two-dimensional (2-D) representation of that environment. This 2-D representation was state-of-the art at one moment in time. Scientists continue to incorporate better data such as the SRTM-90m data and the LIDAR-30m and LIDAR-10m data which shows more terrain features than the SRTM-90m data which was once state of the art. The measurements taken to give us the terrain databases must also be processed to put it on a grid for us to use and these processes become better as new algorithms are implemented. Recently, 3-D representations of terrain have begun being used to help model the losses and will most likely improve the model, but it too will have limitations. For example, it cannot take into account reflections and diffractions from buildings, although the modeling in this area is also improving. It’s biggest limitation at this time is that there is not enough computing power to incorporate all surfaces, all reflections, all diffractions, etc. The world is a very complex place.
The antennas only measured one-polarization of incoming RF-energy. To understand antenna polarization, think of polarized sunglasses. Polarized sunglasses block horizontal polarization by absorbing the horizontally-polarized light energy while allowing the vertically-polarized light energy to pass through. Antennas that would measure all polarizations are difficult to implement in practice.
Here are a few other possible explanations for the differences between the measurement and the model:
The presence of the van distorts the electromagnetic environment compared to what a cellular telephone would do. We performed experiments which showed this was not a huge effect, but it still had some effect.
Weak RF signals are more difficult to measure or capture and this is why sometimes your cellular telephone signal is weak or calls are dropped when your phone is behind a hill, in a downtown area, or in a grove of vegetation.
Atmospheric conditions are not well understood but are known to cause a lot of variations in the area between the transmitting and receiving location. There are ducting effects that occur but are not well understood. These ducting effects allow energy to travel farther than in normal conditions. Usually these effects are found near large bodies of water.
There are more but I’ll stop for now and move onto climate measurements and models.
Climate Measurements and Modeling
Let’s take a look at a couple of maps taken from the Weather Underground website. The first map, shown in Figure 14, was taken on January 13, 2024 in Colorado which was experiencing very cold weather across the state.
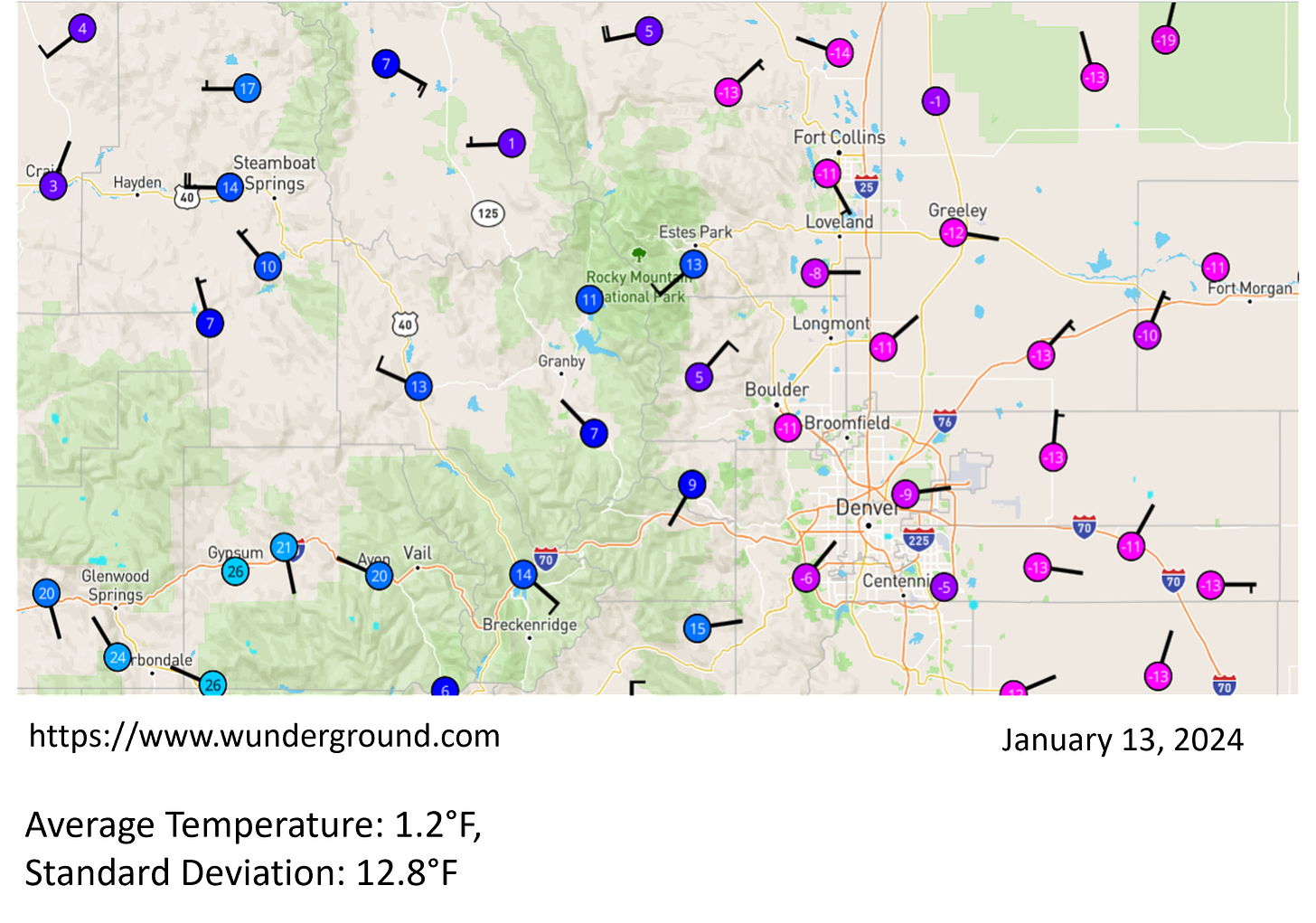
Figure 14 shows many temperature and wind direction readings taken across the state at weather stations on January 13, 2024. We see a high temperature of 26°F in the lower left corner near Glenwood Springs and a low of −19°F in the upper right corner with many readings across the state between those two numbers. There are a total of 47 temperature readings and the average of all those temperatures is 1.2°F. If I gave you that one number, would that tell you anything about the variability of temperatures across the state?
On the same day, I took a screenshot of the temperatures in Hawaii. This map is shown in Figure 15.
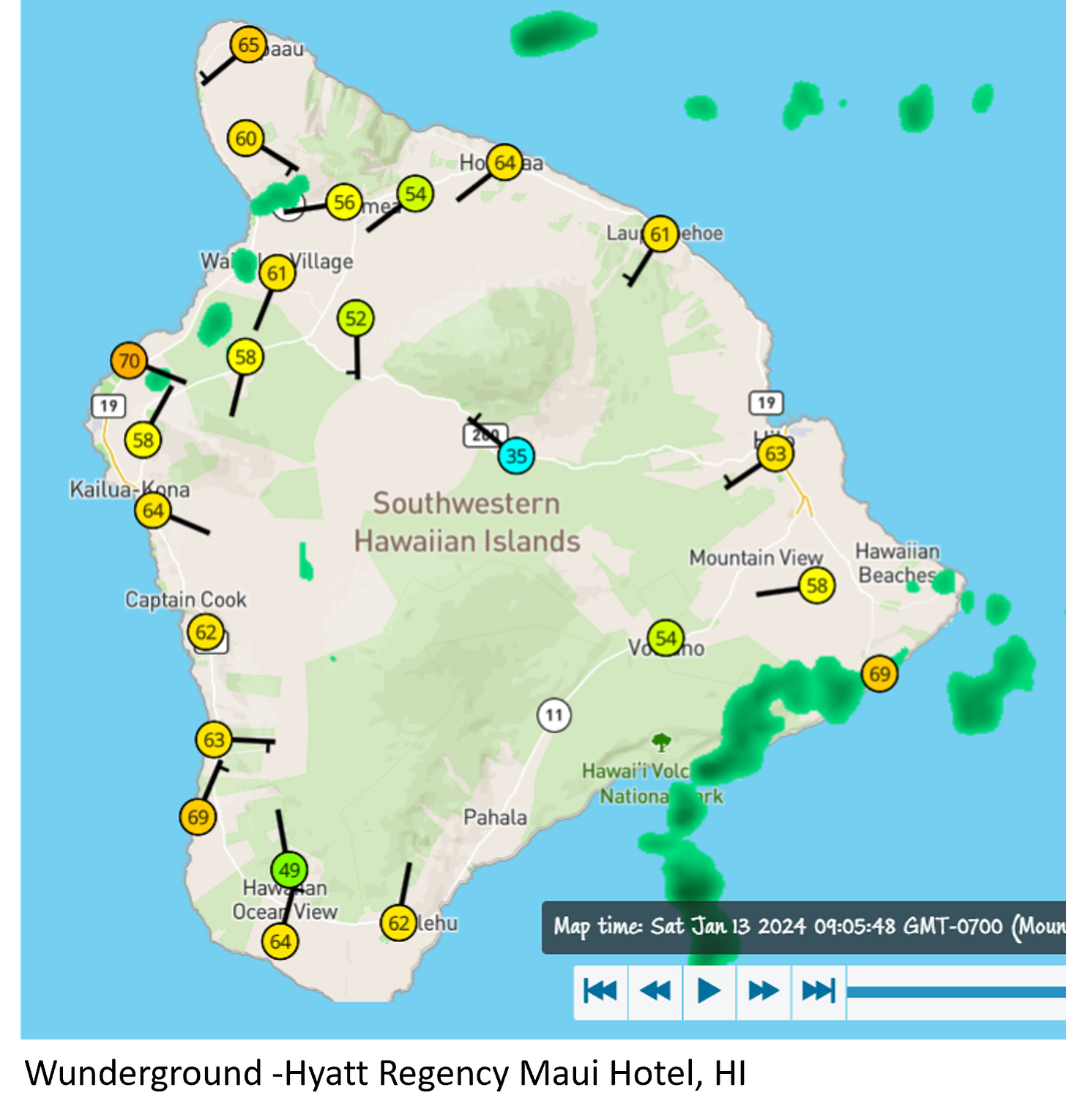
There are a total of 23 temperature readings on the map shown in Figure 15. The average temperature for all temperature readings is 59.6°F. The 35°F temperature reading was taken near the top of the volcano, Mauna Loa, which rises nearly 13,600 feet above sea level. However, most of the temperatures are taken near sea level. We know temperatures decrease with increasing altitude, think about frost on plane window at 30,000 feet. Is it easier to put measurement devices on the ground or up at 30,000 feet? What are we missing if we don’t include temperatures above the ground or the same number of weather stations at higher elevations? Would the average of 59.6°F tell you anything about the temperature at the top of Mauna Loa. Is this average distorted by only one reading at the top of Mauna Loa, or distorted by the numerous other temperature readings at sea level?
When scientists say the global temperature has risen 2°F, how did they get that one number from all the temperature variation we see across the globe, and if the Antarctica is having a particularly cold year, but countries near the equator are having a warm year, does that affect the average? How well does that one number represent all the temperature variations on earth?
Climate Change Model Limitations
Let’s consider just a few limitations that could change the narrative.
More weather stations located taken near the equator where it is warmer
Few temperature readings are taken up through the atmosphere
Weather stations near asphalt and in urban areas where heat is concentrated
Microclimates exist continuously across the earth, climates near bodies of water are different than in mountains or deserts
Cloud cover
Variations in rotation and wobble of the Earth affect solar radiation - Milankovitch Cycle
Weather stations are not all the same and measurement accuracies vary
The dominant green-house gas is water vapor, but we focus on CO2
CO2 shown to lag temperature increases
Conclusion
I have attempted to show that something that has been studied for over one-hundred years like RF propagation still do not take into account many variables that the model must incorporate to make it accurate. Instrumentation is continually improving and the range of energy that they can measure is increasing. The same thing can be said for climate change and our understanding of the mechanisms that produce certain weather effects.
In my two previous substack articles, January Weather Trends in Broomfield, and February Weather Trends in Broomfield, I show that weather predictions over 10 days can change rapidly depending on weather conditions and variables that are still not well understood.
We need to think critically about science and realize that scientific knowledge continuously changes because instrumentation gets better, new experiments are being thought of all the time, and more variables can be added to models to help us understand our observations.
References
(1) Understanding the Impact of Terrain Databases on the Irregular Terrain Model
(2) A High-Performance CW Mobile Channel Sounder
(3) Mobile Channel Sounder Validations Carried Out at the ITS Table Mountain Field Site
(4) Channel Sounder Measurement Verification: Conducted Tests
Fantastic research. Also climate change numbers don't reflect natural climate variability, aka The Sun, only manmade. Does the Sun control climate? Hmmm...do we need a peer-reviewed study to answer that question?